This credential helps organizations identify and develop talent with critical skills for implementing cloud initiatives. Earning AWS Certified Machine Learning – Specialty validates expertise in building, training, tuning, and deploying machine learning (ML) models on AWS.
Who should take this exam?
AWS Certified Machine Learning – Specialty is intended for individuals who perform a development or data science role and have more than one year of experience developing, architecting, or running machine learning/deep learning workloads in the AWS Cloud. Before you take this exam, we recommend you have:
At least two years of hands-on experience developing, architecting, and running ML or deep learning workloads in the AWS Cloud
Ability to express the intuition behind basic ML algorithms
Experience performing basic hyperparameter optimization
Experience with ML and deep learning frameworks
Ability to follow model-training, deployment, and operational best practices
Examkingdom AWS Certified MLS-C01 Exam pdf
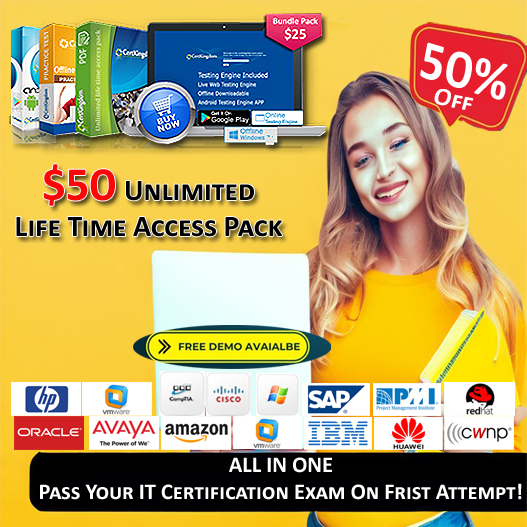
Best AWS Certified MLS-C01 Downloads, AWS Certified MLS-C01 Dumps at Certkingdom.com
Prepare for your exam
You’ve set your goal. Now it’s time to build knowledge and skills to propel your career. Check out these resources from AWS Training and Certification that are relevant to AWS Certified Machine Learning – Specialty. We don’t require that you take any specific training before you take an exam. These recommended resources are opportunities to learn from the experts at AWS.
Additional resources – including AWS Builder Labs and game-based learning to complement your prep – are available with a subscription on AWS Skill Builder.
Looking to become an expert in machine learning? The AWS Certified Machine Learning Specialty (MLS-C01) Certification Practice Exam is the perfect tool to help you achieve your goals. This exam is designed to test your knowledge and skills in machine learning, and is based on the latest industry standards and best practices. With this practice exam, you’ll be able to prepare for the real thing and increase your chances of passing the certification exam on your first try. Get started today and take your machine learning skills to the next level!
AWS Certified Machine Learning Specialty (MLS-C01) Certification Practice Exam is a comprehensive assessment tool designed to evaluate the knowledge and skills of individuals seeking certification in the field of machine learning. This exam is specifically tailored to test the candidate’s proficiency in designing, implementing, and maintaining machine learning solutions using Amazon Web Services (AWS) technologies.
The exam is structured to cover a wide range of topics, including data engineering, exploratory data analysis, modeling, machine learning algorithms, and deployment and operationalize of machine learning models. The exam is designed to test the candidate’s ability to apply these concepts in real-world scenarios, and to evaluate their understanding of the best practices and principles of machine learning.
AWS Certified Machine Learning Specialty (MLS-C01) Certification Practice Exam is a comprehensive and reliable resource designed to help individuals prepare for the AWS Certified Machine Learning Specialty (MLS-C01) certification exam. This practice exam is specifically tailored to provide candidates with a realistic and challenging experience that closely mirrors the actual certification exam.
The practice exam is comprised of multiple-choice questions that cover a wide range of topics related to machine learning on the AWS platform. These topics include data engineering, exploratory data analysis, modeling, machine learning algorithms, and more. Each question is carefully crafted to test the candidate’s knowledge and understanding of the subject matter, as well as their ability to apply that knowledge in real-world scenarios.
AWS Certified Machine Learning Specialty (MLS-C01) Certification Practice Exam is an ideal tool for individuals who are looking to enhance their skills and knowledge in the field of machine learning, as well as those who are seeking to obtain the AWS Certified Machine Learning Specialty (MLS-C01) certification. With its comprehensive coverage, challenging questions, and realistic exam experience, this practice exam is an essential resource for anyone looking to succeed in the field of machine learning on the AWS platform.
AWS Certified Machine Learning Specialty (MLS-C01) Certification Practice Exam is an essential tool for individuals seeking to validate their expertise in machine learning and AWS technologies. It provides a rigorous and comprehensive assessment of the candidate’s knowledge and skills, and serves as a valuable credential for professionals seeking to advance their careers in this rapidly growing field. With its focus on practical application and real-world scenarios, this exam is an indispensable resource for anyone seeking to demonstrate their proficiency in machine learning and AWS technologies.
AWS Certified Machine Learning – Specialty Exam details:
Exam Name : AWS machine learning specialty
Exam code: MLS-C01
Exam voucher cost: $300 USD
Exam languages: English, Japanese, Korean, and Simplified Chinese
Exam format: Multiple-choice, multiple-answer
Number of questions: 65 (estimate)
Length of exam: 170 minutes
Passing grade: Score is from 750-1000, passing grade of 750
AWS certifications are valid for 3 years, after which you must recertify (you get a 50% off voucher for your recertification exam from AWS)
AWS Machine Learning Certification Syllabus
Data Engineering: 20%
Exploratory Data Analysis: 24%
Modeling: 36%
Machine Learning Implementation and Operations: 20%
# Domain 1: Data Engineering
The sections that are tested and covered in this domain are as follows:
Development of Machine Learning repositories
Implementation and identification of data ingestion and transformation solutions
# Domain 2: Exploratory Data Analysis
This module comprises modeling and other Machine Learning concepts, along with the ones mentioned below:
Preparation and sanitization of data for modeling
Feature engineering
Data visualization and analyzing for Machine Learning
# Domain 3: Modeling
This is among the most vital domain among the rest when it comes to preparation and examination. The sections covered in this domain are listed below:
Relating business issues with Machine Learning
Training Machine Learning models
Identification of the right model for the respective Machine Learning business problem
Hyperparameter optimization
Evaluation of Machine Learning models
# Domain 4: Machine Learning Implementation and Operations
This domain of the syllabus includes concepts of Implementation and services of Machine Learning, some of which include:
Development of Machine Learning solutions for availability, resiliency, fault-tolerance, and performance
Recommendation and implementation of the right Machine Learning services for the respective issues
Application of basic security practices of AWS to Machine Learning solutions
AWS Certified Machine Learning – Specialty Certification Practice Exam Benefits:
AWS Certified Machine Learning – Specialty Certification Practice Exam is a comprehensive and reliable tool designed to help individuals prepare for the AWS Certified Machine Learning – Specialty certification exam. This practice exam offers a range of benefits, including the opportunity to assess one’s knowledge and skills in the field of machine learning, identify areas of strength and weakness, and gain confidence in tackling the actual certification exam.
The practice exam is designed to simulate the actual certification exam, with a similar format, structure, and level of difficulty. It covers a wide range of topics, including data engineering, exploratory data analysis, modeling, machine learning algorithms, and deployment and implementation. The questions are carefully crafted to test one’s understanding of the concepts and principles of machine learning, as well as their ability to apply them in real-world scenarios.
AWS Certified Machine Learning – Specialty Certification Practice Exam is also a valuable resource for those seeking to enhance their professional credentials and advance their career in the field of machine learning. By passing the certification exam, individuals can demonstrate their expertise and proficiency in the latest machine learning technologies and techniques, and gain recognition from employers and peers alike.
AWS Certified Machine Learning – Specialty Certification Practice Exam is an essential tool for anyone looking to achieve AWS certification in machine learning. With its comprehensive coverage, realistic format, and valuable benefits, it is the ideal resource for preparing for the certification exam and achieving success in the field of machine learning.
Who this course is for:
You Will Be Able To Zero to Hero in AWS Certified Machine Learning Specialty.
Basic to Advance AWS Certified Machine Learning Specialty Step by Step.
Have a fundamental understanding of the AWS Certified Machine Learning Specialty Certification.
By taking this course, develop your AWS Certified Machine Learning Specialty.
Prepare for the AWS Certified Machine Learning Specialty Exam.
Passing the AWS Certified Machine Learning Specialty certification exam with confidence.
Passing the AWS Certified Machine Learning Specialty certification exam on your first attempt.
Anyone who is looking to PASS the AWS Certified Machine Learning Specialty exam.
Students who wish to sharpen their knowledge of AWS Certified Machine Learning Specialty.
Students who are preparing for AWS Certified Machine Learning Specialty.
AWS Certified Machine Learning Specialty skills to your resume.
Anyone looking forward to brush up their skills.
Sample Question and Answers
QUESTION 1
A Machine Learning Specialist is working with multiple data sources containing billions of records
that need to be joined. What feature engineering and model development approach should the
Specialist take with a dataset this large?
A. Use an Amazon SageMaker notebook for both feature engineering and model development
B. Use an Amazon SageMaker notebook for feature engineering and Amazon ML for model development
C. Use Amazon EMR for feature engineering and Amazon SageMaker SDK for model development
D. Use Amazon ML for both feature engineering and model development.
Answer: C
Explanation:
Amazon EMR is a service that can process large amounts of data efficiently and cost-effectively. It
can run distributed frameworks such as Apache Spark, which can perform feature engineering on big
data. Amazon SageMaker SDK is a Python library that can interact with Amazon SageMaker service to
train and deploy machine learning models. It can also use Amazon EMR as a data source for training data.
References:
Amazon EMR
Amazon SageMaker SDK
QUESTION 2
A Machine Learning Specialist has completed a proof of concept for a company using a small data
sample and now the Specialist is ready to implement an end-to-end solution in AWS using Amazon
SageMaker The historical training data is stored in Amazon RDS
Which approach should the Specialist use for training a model using that data?
A. Write a direct connection to the SQL database within the notebook and pull data in
B. Push the data from Microsoft SQL Server to Amazon S3 using an AWS Data Pipeline and provide the S3 location within the notebook.
C. Move the data to Amazon DynamoDB and set up a connection to DynamoDB within the notebook to pull data in
D. Move the data to Amazon ElastiCache using AWS DMS and set up a connection within the notebook to pull data in for fast access.
Answer: B
Explanation:
Pushing the data from Microsoft SQL Server to Amazon S3 using an AWS Data Pipeline and providing
the S3 location within the notebook is the best approach for training a model using the data stored in
Amazon RDS. This is because Amazon SageMaker can directly access data from Amazon S3 and train
models on it. AWS Data Pipeline is a service that can automate the movement and transformation of
data between different AWS services. It can also use Amazon RDS as a data source and Amazon S3 as
a data destination. This way, the data can be transferred efficiently and securely without writing any
code within the notebook. References:
Amazon SageMaker
AWS Data Pipeline
QUESTION 3
Which of the following metrics should a Machine Learning Specialist generally use to
compare/evaluate machine learning classification models against each other?
A. Recall
B. Misclassification rate
C. Mean absolute percentage error (MAPE)
D. Area Under the ROC Curve (AUC)
Answer: D
Explanation:
Area Under the ROC Curve (AUC) is a metric that measures the performance of a binary classifier
across all possible thresholds. It is also known as the probability that a randomly chosen positive
example will be ranked higher than a randomly chosen negative example by the classifier. AUC is a
good metric to compare different classification models because it is independent of the class
distribution and the decision threshold. It also captures both the sensitivity (true positive rate) and
the specificity (true negative rate) of the model. References:
AWS Machine Learning Specialty Exam Guide
AWS Machine Learning Specialty Sample Questions
QUESTION 4
A Machine Learning Specialist is using Amazon Sage Maker to host a model for a highly available
customer-facing application.
The Specialist has trained a new version of the model, validated it with historical data, and now
wants to deploy it to production To limit any risk of a negative customer experience, the Specialist
wants to be able to monitor the model and roll it back, if needed
What is the SIMPLEST approach with the LEAST risk to deploy the model and roll it back, if needed?
A. Create a SageMaker endpoint and configuration for the new model version. Redirect production
traffic to the new endpoint by updating the client configuration. Revert traffic to the last version if
the model does not perform as expected.
B. Create a SageMaker endpoint and configuration for the new model version. Redirect production
traffic to the new endpoint by using a load balancer Revert traffic to the last version if the model
does not perform as expected.
C. Update the existing SageMaker endpoint to use a new configuration that is weighted to send 5%
of the traffic to the new variant. Revert traffic to the last version by resetting the weights if the model
does not perform as expected.
D. Update the existing SageMaker endpoint to use a new configuration that is weighted to send
100% of the traffic to the new variant Revert traffic to the last version by resetting the weights if the
model does not perform as expected.
Answer: C
Explanation:
Updating the existing SageMaker endpoint to use a new configuration that is weighted to send 5% of
the traffic to the new variant is the simplest approach with the least risk to deploy the model and roll
it back, if needed. This is because SageMaker supports A/B testing, which allows the Specialist to
compare the performance of different model variants by sending a portion of the traffic to each
variant. The Specialist can monitor the metrics of each variant and adjust the weights accordingly. If
the new variant does not perform as expected, the Specialist can revert traffic to the last version by
resetting the weights to 100% for the old variant and 0% for the new variant. This way, the Specialist
can deploy the model without affecting the customer experience and roll it back easily if
needed. References:
Amazon SageMaker
Deploying models to Amazon SageMaker hosting services
QUESTION 5
A manufacturing company has a large set of labeled historical sales data The manufacturer would like
to predict how many units of a particular part should be produced each quarter Which machine
learning approach should be used to solve this problem?
A. Logistic regression
B. Random Cut Forest (RCF)
C. Principal component analysis (PCA)
D. Linear regression
Answer: D
Explanation:
Linear regression is a machine learning approach that can be used to solve this problem. Linear
regression is a supervised learning technique that can model the relationship between one or more
input variables (features) and an output variable (target). In this case, the input variables could be
the historical sales data of the part, such as the quarter, the demand, the price, the inventory, etc.
The output variable could be the number of units to be produced for the part. Linear regression can
learn the coefficients (weights) of the input variables that best fit the output variable, and then use
them to make predictions for new data. Linear regression is suitable for problems that involve
continuous and numeric output variables, such as predicting house prices, stock prices, or sales
volumes. References:
AWS Machine Learning Specialty Exam Guide
Linear Regression
QUESTION 6
A manufacturing company has structured and unstructured data stored in an Amazon S3 bucket A
Machine Learning Specialist wants to use SQL to run queries on this data.
Which solution requires the LEAST effort to be able to query this data?
A. Use AWS Data Pipeline to transform the data and Amazon RDS to run queries.
B. Use AWS Glue to catalogue the data and Amazon Athena to run queries
C. Use AWS Batch to run ETL on the data and Amazon Aurora to run the quenes
D. Use AWS Lambda to transform the data and Amazon Kinesis Data Analytics to run queries
Answer: B
Explanation:
AWS Glue is a serverless data integration service that can catalogue, clean, enrich, and move data
between various data stores. Amazon Athena is an interactive query service that can run SQL queries
on data stored in Amazon S3. By using AWS Glue to catalogue the data and Amazon Athena to run
queries, the Machine Learning Specialist can leverage the existing data in Amazon S3 without any
additional data transformation or loading. This solution requires the least effort compared to the
other options, which involve more complex and costly data processing and storage
services. References: AWS Glue, Amazon Athena
QUESTION 7
A Machine Learning Specialist is packaging a custom ResNet model into a Docker container so the
company can leverage Amazon SageMaker for training The Specialist is using Amazon EC2 P3
instances to train the model and needs to properly configure the Docker container to leverage the NVIDIA GPUs
What does the Specialist need to do1?
A. Bundle the NVIDIA drivers with the Docker image
B. Build the Docker container to be NVIDIA-Docker compatible
C. Organize the Docker container’s file structure to execute on GPU instances.
D. Set the GPU flag in the Amazon SageMaker Create TrainingJob request body
Answer: B
Explanation:
To leverage the NVIDIA GPUs on Amazon EC2 P3 instances, the Machine Learning Specialist needs to
build the Docker container to be NVIDIA-Docker compatible. NVIDIA-Docker is a tool that enables
GPU-accelerated containers to run on Docker. It automatically configures the container to access the
NVIDIA drivers and libraries on the host system. The Specialist does not need to bundle the NVIDIA
drivers with the Docker image, as they are already installed on the EC2 P3 instances. The Specialist
does not need to organize the Docker container’s file structure to execute on GPU instances, as this is
not relevant for GPU compatibility. The Specialist does not need to set the GPU flag in the Amazon
SageMaker Create TrainingJob request body, as this is only required for using Elastic Inference
accelerators, not EC2 P3 instances. References: NVIDIA-Docker, Using GPU-Accelerated
Containers, Using Elastic Inference in Amazon SageMaker
QUESTION 8
A large JSON dataset for a project has been uploaded to a private Amazon S3 bucket The Machine
Learning Specialist wants to securely access and explore the data from an Amazon SageMaker
notebook instance A new VPC was created and assigned to the Specialist
How can the privacy and integrity of the data stored in Amazon S3 be maintained while granting
access to the Specialist for analysis?
A. Launch the SageMaker notebook instance within the VPC with SageMaker-provided internet
access enabled Use an S3 ACL to open read privileges to the everyone group
B. Launch the SageMaker notebook instance within the VPC and create an S3 VPC endpoint for the
notebook to access the data Copy the JSON dataset from Amazon S3 into the ML storage volume on
the SageMaker notebook instance and work against the local dataset
C. Launch the SageMaker notebook instance within the VPC and create an S3 VPC endpoint for the
notebook to access the data Define a custom S3 bucket policy to only allow requests from your VPC
to access the S3 bucket
D. Launch the SageMaker notebook instance within the VPC with SageMaker-provided internet
access enabled. Generate an S3 pre-signed URL for access to data in the bucket
Answer: C
Explanation:
The best way to maintain the privacy and integrity of the data stored in Amazon S3 is to use a
combination of VPC endpoints and S3 bucket policies. A VPC endpoint allows the SageMaker
notebook instance to access the S3 bucket without going through the public internet. A bucket policy
allows the S3 bucket owner to specify which VPCs or VPC endpoints can access the bucket. This way,
the data is protected from unauthorized access and tampering. The other options are either insecure
(A and D) or inefficient (B). References: Using Amazon S3 VPC Endpoints, Using Bucket Policies and User Policies
QUESTION 9
Given the following confusion matrix for a movie classification model, what is the true class
frequency for Romance and the predicted class frequency for Adventure?
A. The true class frequency for Romance is 77.56% and the predicted class frequency for Adventure is 20 85%
B. The true class frequency for Romance is 57.92% and the predicted class frequency for Adventure is 1312%
C. The true class frequency for Romance is 0 78 and the predicted class frequency for Adventure is (0 47 – 0.32).
D. The true class frequency for Romance is 77.56% * 0.78 and the predicted class frequency for Adventure is 20 85% ‘ 0.32
Answer: B
Explanation:
The true class frequency for Romance is the percentage of movies that are actually Romance out of
all the movies. This can be calculated by dividing the sum of the true values for Romance by the total
number of movies. The predicted class frequency for Adventure is the percentage of movies that are
predicted to be Adventure out of all the movies. This can be calculated by dividing the sum of the
predicted values for Adventure by the total number of movies. Based on the confusion matrix, the
true class frequency for Romance is 57.92% and the predicted class frequency for Adventure is
13.12%. References: Confusion Matrix, Classification Metrics
QUESTION 10
A Machine Learning Specialist is building a supervised model that will evaluate customers’
satisfaction with their mobile phone service based on recent usage The model’s output should infer
whether or not a customer is likely to switch to a competitor in the next 30 days
Which of the following modeling techniques should the Specialist use1?
A. Time-series prediction
B. Anomaly detection
C. Binary classification
D. Regression
Answer: C
Explanation:
The modeling technique that the Machine Learning Specialist should use is binary classification.
Binary classification is a type of supervised learning that predicts whether an input belongs to one of
two possible classes. In this case, the input is the customer’s recent usage data and the output is
whether or not the customer is likely to switch to a competitor in the next 30 days. This is a binary
outcome, either yes or no, so binary classification is suitable for this problem. The other options are
not appropriate for this problem. Time-series prediction is a type of supervised learning that
forecasts future values based on past and present data. Anomaly detection is a type of unsupervised
learning that identifies outliers or abnormal patterns in the data. Regression is a type of supervised
learning that estimates a continuous numerical value based on the input features. References: Binary
Classification, Time Series Prediction, Anomaly Detection, Regression